

#Download highfive apk
Once the download is complete, you can find the APK in "Downloads" section in your browser.īefore you can install it on your phone you will need to make sure that third-party apps are allowed on your device. You can download Highfive Video Conferencing APK by clicking the above button and that will initiate a download.
#Download highfive how to
How to install Highfive Video Conferencing APK from your android phone? We have added a button above to download Highfive Video Conferencing official app file.Īlways download android from Google Play store, unless they don't have the app you're looking for. You can download any android app's APK from many sources such as ApkMirror, ApkPure etc.īut we strongly recommend not to download from any third-party sources. The experimental results demonstrated the superior efficiency.Where can I download Highfive Video Conferencing APK file? The proposed method was tested and evaluated on the WEB Interaction dataset and the UT interaction dataset. Classification was achieved using multiple instance learning (MIL), which was more suitable for complex environments. After that, an over-complete dictionary was learned with the descriptors and all the descriptors were encoded using sparse coding (SC). In this way, the discriminative descriptors could be extracted, which was also an effective solution to the problem that the description of the feature second-order statistics is insufficient. Then, the trajectory tunnels were characterized by means of feature covariance matrices. Firstly, the dense trajectories (DT) extracted from the video were clustered into different groups to eliminate the irrelevant trajectories, which could greatly reduce the noise influence on feature extraction.
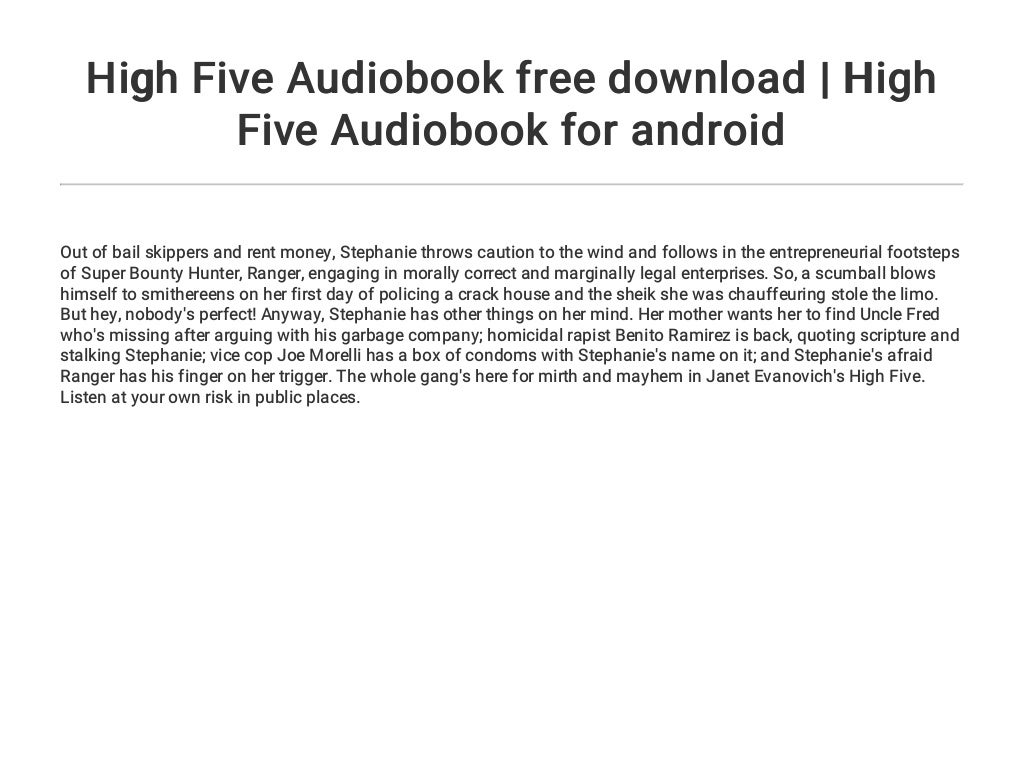
Experimental results on the UT-Interaction dataset show that the method achieved better recognition performance with simple implementation.Ī new method for interaction recognition based on sparse representation of feature covariance matrices was presented.
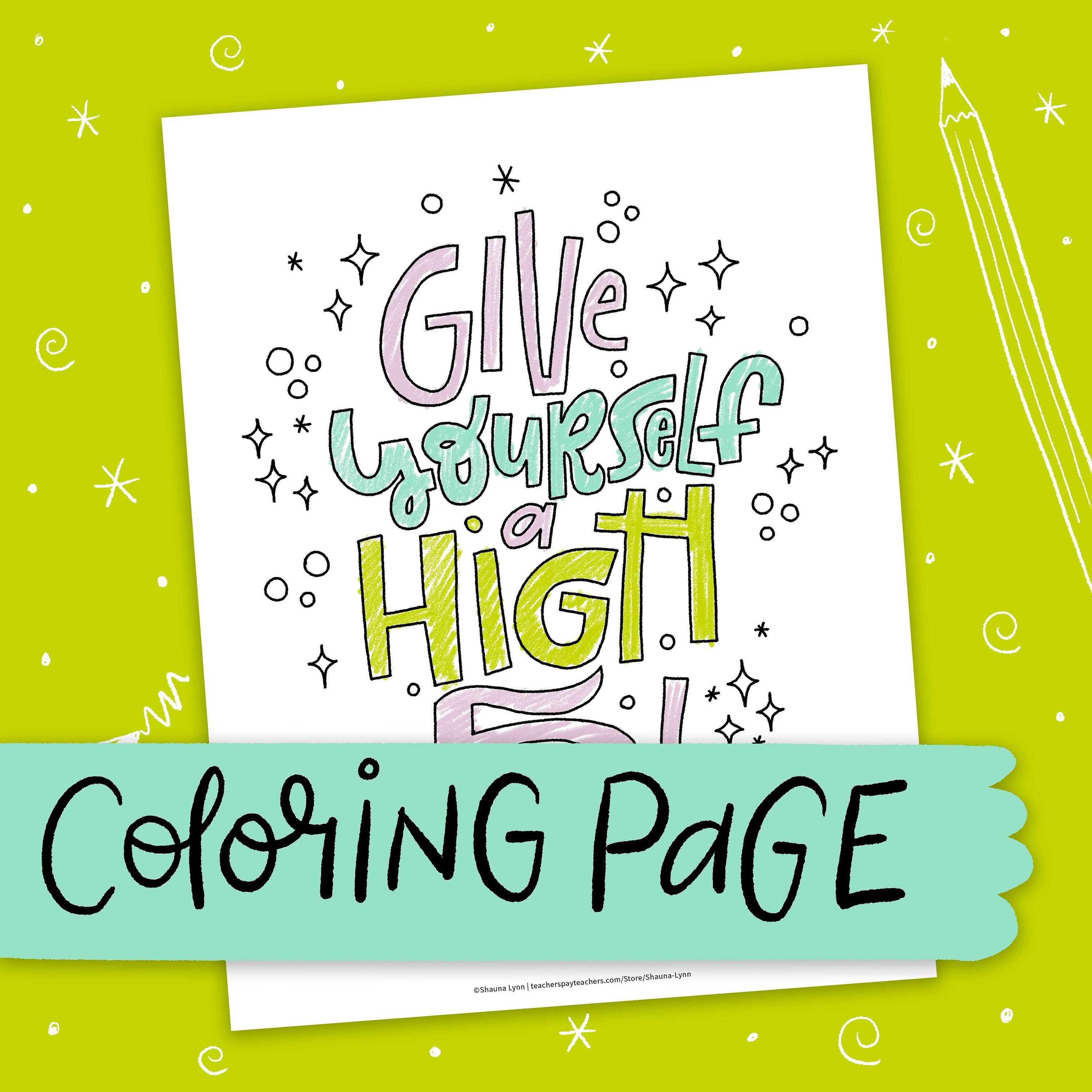
Finally, HMM method was utilized to model and recognize the human interactions. Then the k-means algorithm was utilized to build the bag of visual words (BOVW) with HOG feature from all the training videos, and each frame in a video was described by co-occurring visual matrix with BOVW, and the video was represented by the co-occurring visual matrix sequence. In the individual segmentation framework, ROI was firstly extracted by frame difference and the distance analysis between two interacting persons, and segmented into two separate interacting persons with prior knowledge, such as color and body outline. An innovative and effective way based on the co-occurring visual matrix sequence was proposed to improve the accuracy in this paper, which sufficiently utilized the superiority of co-occurring visual matrix and probability graph model. However this kind of methods has relatively low recognition accuracy. The human interaction recognition methods based on motion co-occurrence have been an efficient solution for its reasonable expression and simple operation. Experiments on the well-known UT-Interaction dataset have demonstrated the performance of our approach by comparison with state-of-art methods. Our model is computationally efficient and can be trained on a small training dataset while maintaining a good generalization and interpretation capabilities. These weights are automatically estimated during GFR-MKLR training using gradient descent minimisation. We encode group sparsity in GFR-MKLR through relevance weights reflecting each group (gesture) discrimination capability between different interaction categories. The groups consist of motion features extracted from tracking interacting persons joints over time. The group structure in GFR-MKLR is chosen to reflect a representation of interactions at the level of gestures, which ensures more robustness to intra-class variability due to occlusions and changes in subject appearance, body size and viewpoint. Our approach couples kernel and group sparsity modelling to ensure highly precise interaction classification. We propose an approach for human interaction recognition (HIR) in videos using multinomial kernel logistic regression with group-of-features relevance (GFR-MKLR).
